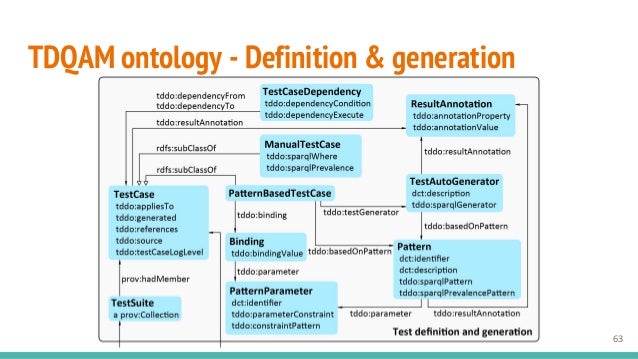
Oct 16, · @universityofky posted on their Instagram profile: “Like her sticker says, “Find your people.” College is a great place to do just that. Tag “your ” Most of them are native speakers and PhD holders able to take care of any assignment you need help with. M. original pages written. Each paper is composed from scratch to meet your assignment instructions. We then use a plagiarism-detection software to ensure that it is, actually, completely plagiarism free. We ensure that there is no way This is a difficult question: feature extraction is one solution the other is to use image correlation. Correlation works for signal processing, But the simplest approach is to make an
UCI Machine Learning Repository: Iris Data Set
Iris Data Set Download : Data FolderData Set Description. Creator: R. This is perhaps the best known database to be found in the pattern recognition literature. Fisher's paper is a classic in the field and is referenced frequently to this day, phd thesis feature extraction. The data set contains 3 classes of 50 instances each, where each class refers to a type of iris plant.
One class is linearly separable from the other phd thesis feature extraction the latter are NOT linearly separable from each other. Predicted attribute: class of iris plant. This is an exceedingly simple domain. This data differs from the data presented in Fishers article identified by Steve Chadwick, spchadwick ' ' espeedaz. The 35th sample should be: 4. The 38th sample: 4. sepal length in cm 2.
sepal width in cm 3. petal length in cm 4. petal width in cm 5. class: -- Iris Setosa -- Iris Versicolour -- Iris Virginica. ISBN See page IEEE Transactions on Pattern Analysis and Machine Intelligence, Vol. PAMI-2, No. IEEE Transactions on Information Theory, May Manuel Oliveira. Library Release Form Name of Author: Stanley Robson de Medeiros Oliveira Title of Thesis: Data Transformation For Privacy-Preserving Data Mining Degree: Doctor of Philosophy Year this Degree Granted.
University of Alberta Library. Ping Zhong and Masao Fukushima. A Regularized Nonsmooth Newton Method for Multi-class Support Vector Machines. Anthony K H Tung and Xin Xu and Beng Chin Ooi. CURLER: Finding and Visualizing Nonlinear Correlated Clusters. SIGMOD Conference. Igor Fischer and Jan Poland. Amplifying the Block Matrix Structure for Spectral Clustering. Telecommunications Lab.
Sotiris B. Kotsiantis and Panayiotis E. Logitboost phd thesis feature extraction Simple Bayesian Classifier. Sugato Basu. Semi-Supervised Clustering with Limited Background Knowledge. Judith E. Devaney and Steven G. Satterfield and John G. Hagedorn and John T. Kelso and Adele P. Peskin and William George and Terence J. Griffin and Howard K. Hung and Ronald D. Science at the Speed of Thought. Ambient Intelligence for Scientific Discovery.
Jennifer G. Dy and Carla Brodley. Feature Selection for Unsupervised Learning. Journal of Machine Learning Research, phd thesis feature extraction, 5. Phd thesis feature extraction Eggermont and Joost N.
Kok and Walter A. Genetic Programming for data classification: partitioning the search space. Remco R. Bouckaert and Eibe Frank. Evaluating the Replicability of Significance Tests for Comparing Learning Algorithms. Mikhail Bilenko and Sugato Basu and Raymond J. Integrating constraints and metric learning in semi-supervised clustering.
Qingping Tao Ph. Yuan Jiang and Zhi-Hua Zhou. Phd thesis feature extraction Training Data for kNN Classifiers with Neural Network Ensemble. ISNN 1. Manoranjan Dash and Huan Liu and Peter Scheuermann and Kian-Lee Tan. Fast hierarchical clustering and its validation. Data Knowl. Eng, Bob Ricks and Dan Ventura. Training a Quantum Neural Network. Eibe Frank and Mark Hall. Visualizing Class Probability Estimators.
Ross J. Micheals and Patrick Grother and P. Jonathon Phillips. The NIST HumanID Evaluation Framework. Also Appears as Technical Report, UT-AI. PhD Proposal. Dick de Ridder and Olga Kouropteva and Oleg Okun and Matti Pietikäinen and Robert P W Duin. Supervised Locally Linear Embedding.
Aristidis Likas and Nikos A. Vlassis and Jakob J. The global k-means clustering algorithm. Pattern Recognition, Zhi-Hua Zhou and Yuan Jiang and Shifu Chen. Extracting symbolic rules from trained neural network ensembles, phd thesis feature extraction. AI Commun, Jeremy Kubica and Andrew Moore. Probabilistic Noise Identification and Data Cleaning. Julie Greensmith. New Frontiers For An Artificial Immune System. Digital Media Systems Laboratory HP Laboratories Bristol.
Geoffrey Holmes and Bernhard Pfahringer and Richard Kirkby and Eibe Frank and Mark A. Multiclass Alternating Decision Trees. Inderjit S. Dhillon and Dharmendra S. Modha and W. Scott Spangler. Class visualization of high-dimensional data with applications.
Department of Computer Sciences, University of Texas.
PhD Thesis Defense: Manuel A. Florez Torres: A Global Study of Double Seismic Zones
, time: 1:03:55Online MA in Strategic Intelligence & Analysis | Northeastern University
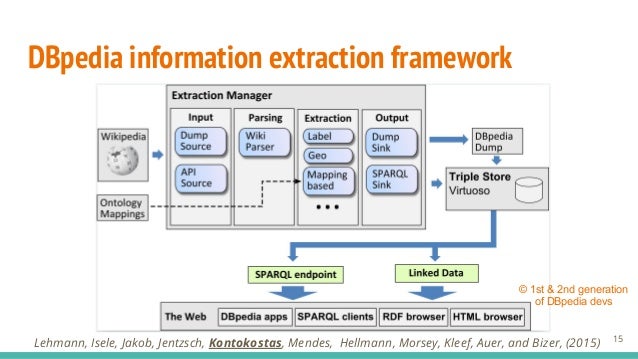
Oct 16, · @universityofky posted on their Instagram profile: “Like her sticker says, “Find your people.” College is a great place to do just that. Tag “your ” About. Dr. Mohit Bansal is the John R. & Louise S. Parker Associate Professor and the Director of the MURGe-Lab (in the UNC-NLP Group) in the Computer Science department at the University of North Carolina (UNC) Chapel blogger.com to this, he was a research assistant professor (3-year endowed position) at blogger.com received his Ph.D. in from the University of California at Berkeley This is a difficult question: feature extraction is one solution the other is to use image correlation. Correlation works for signal processing, But the simplest approach is to make an
No comments:
Post a Comment